The Uncharted Potential of Large Language Models (LLMs): A Cautiously Optimistic Perspective
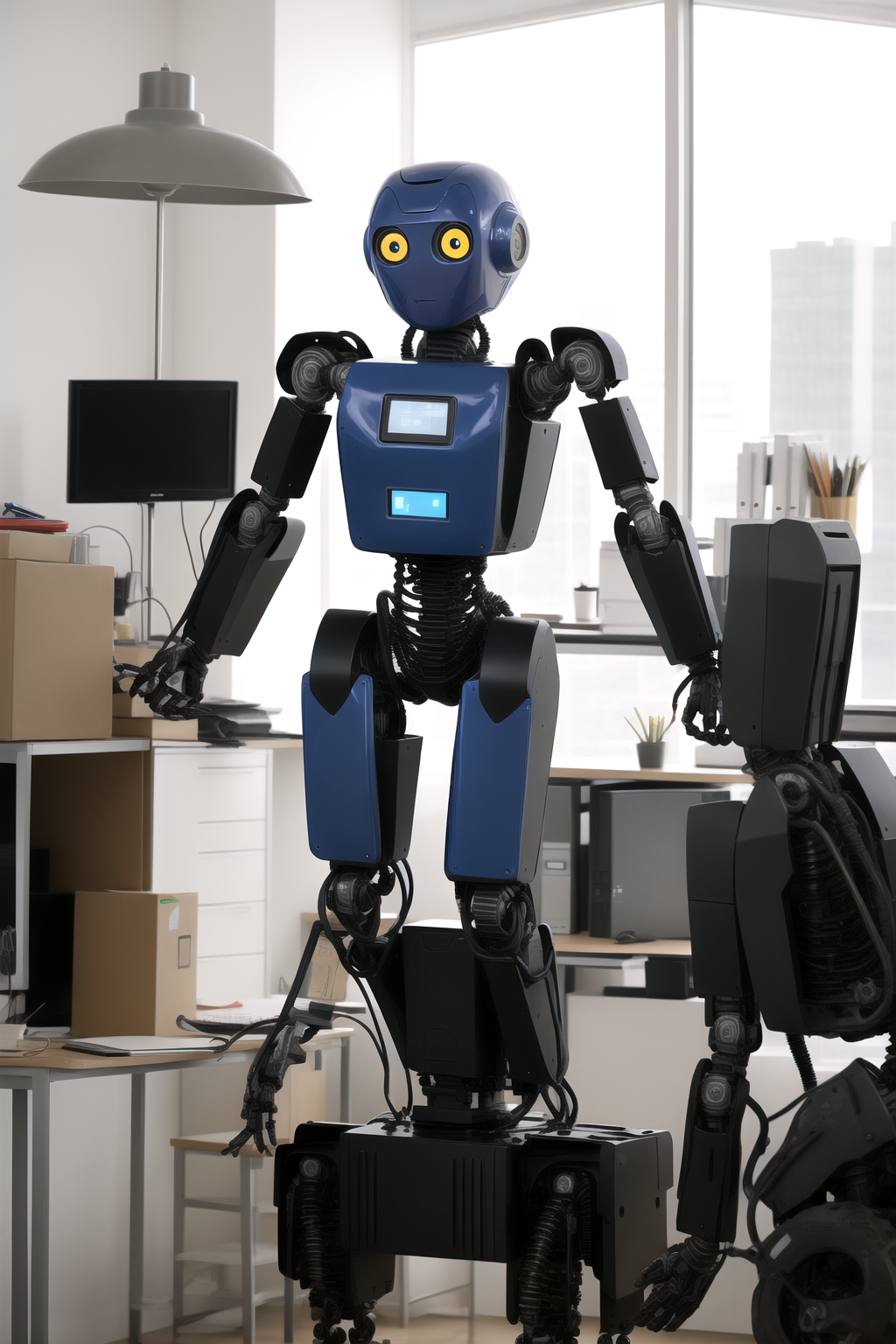
The hype around Large Language Models (LLMs) may not have reached its peak yet, but the potential of these AI agents is undeniable. The integration of LLMs into various sectors is still in its nascent stages, and while there are challenges to overcome, the future looks promising.
Generative AI, which includes LLMs, presents unique opportunities for enterprises. These models can create new services and solutions, allowing technology leaders to enter new markets and gain market share. However, the technology also comes with its own set of challenges. For instance, proprietary data can be exposed to competitors, and there are potential reputational or operational risks due to the models' bias or hallucinations.
Large enterprises would be wise to build or optimize one or more generative AI models specific to their business requirements within the next few years. Don't be square and get left behind. Companies like Bloomberg are already generating world-class performance by building their own generative AI tools leveraging internal data.
However, building enterprise generative AI models comes with its own set of guidelines. The models should be consistent, controlled, ethically trained, explainable, fair, licensed, secure, and private. Achieving these goals can be challenging, but they are necessary for the successful implementation of generative AI without getting bad PR.
There are three main approaches to building an enterprise's LLM infrastructure in a controlled environment: Build Your Own Model (BYOM), fine-tuning, and Reinforcement Learning from Human Feedback (RLHF). Each approach has its own advantages and drawbacks, and the choice depends on the specific needs and resources of the enterprise.
Several machine learning platforms have released foundation models with commercial licenses that can be used as base models to build enterprise large language models. These include Llama 2 by Meta, Falcon LLM by Technology Innovation Institute (TII) in Abu Dhabi, Dolly 2.0 by Databricks, and others.
The right tech stack for building large language models includes Machine Learning Operations (MLOps) platforms, Large Language Model Operations (LLMOps) tools and frameworks, and AI risk management tools. It's also crucial to have a robust data infrastructure.
Evaluating the performance of large models is a complex task due to issues in benchmark datasets and inconsistency of human reviews. An iterative approach that increases investment in the evaluation as models get closer to being used in production is recommended.
In conclusion, while the hype around LLMs aiding Skynet is overstated, the potential of these AI agents is immense. With the right approach, guidelines, and tools, enterprises can leverage the power of LLMs to revolutionize their operations and services. The journey may be challenging, but the destination promises to be worth it. So, let's tread this path with cautious optimism.
There is a great ecosystem of proprietary and open-source LLM tech out there. We have decided that you can try them all! Check out our chatbot that allows you to swap out the model driving the chat with any browser capable of installing Chrome extensions.